GenAI
Sustainable Computing
How to reduce the environmental impact of GenAI
The emergence of generative AI (GenAI) and large language models (LLMs) has now enabled several industries to revolutionize how they process and generate data. However, these innovations come with significant computational requirements. These costs are not only monetary but also environmental. According to a Harvard Business Review article, the data center industry, of which the GenAI field relies on, contributes to around 2–3% of global greenhouse emissions. These industries require a large amount of electricity and water. An article in the Washington Post details how data centers are among the top 10 most water-consumptive commercial industries in the United States.
Given these figures, we should be mindful of the costs and consequences of running our GenAI models. In this article, we will discuss some of the issues and methods to keep in mind in order to reduce the environmental impact of your GenAI usage.
Opt for smaller models
It’s not necessary to use the latest and greatest LLM. In many instances, a smaller model, sometimes called a small language model (SLM) is perfectly viable. Not only are SLMs more cost effective, they’re also less costly for the environment as they require less computational power. LLMs and conversational AIs such as GPT-4 and Mistral are extremely powerful. Even though significantly smaller, the BERT model is perfectly capable as well. If you want to learn more about how you can use SLMs such as BERT, read UbiOps’ article detailing 6 applications of LLMs.
Fine-tune or use already-trained models
A significant environmental cost when it comes to AI models are their training processes. According to an MIT Technology Review article, taking BLOOM as an example, its training process produced around 25 metric tons of CO2, which rose to 50 if the manufacturing of the equipment was taken into account. To give some perspective, 1 ton of Co2 is equivalent to the amount produced by a 2,500 mile car ride, which is around the distance it takes to drive from Amsterdam in The Netherlands to Tbilisi in Georgia. Given this, it is far more environmentally friendly to use an already trained model.
Alternatively, if you really need a model customized for your use case, you can fine-tune an already-trained model by retraining it on some specific data. Fine-tuning can reportedly be 10–100 times more energy efficient than training a model from scratch.
Use a sustainable cloud and computing provider
However, when it comes to GenAI, large computational needs are unavoidable. Therefore, you should use a computing provider who takes environmental factors into consideration.
Innovative cooling
Cooling is an essential part of increasing a data center’s efficiency when performing computational tasks. Computation and storage hardware need to be prevented from overheating in order to keep them functioning normally and extend their lifetime. Bytesnet uses closed-loop liquid cooling techniques for many systems, which lowers water and energy consumption as compared to traditional cooling techniques. Furthermore, some Bytesnet’s IT equipment is submerged in a special oil which increases cooling effectiveness, therefore lowering the amount of energy needed.
Recycling heat
As mentioned above, data centers are constantly generating heat. Instead of letting that heat into the atmosphere, you could reuse it for other purposes. Bytesnet uses the heat generated by its d’Root data center to help warm the city of Groningen through WarmteStad. This reduces the amount of overall energy needed by recycling available heat.
Green energy
To offset the high energy consumption, many data centers like Bytesnet have now transitioned to sustainable energy sources. This can be done by purchasing energy which has a Guarantee of Origin (GoO). A GoO certificate is proof that energy has come from a sustainable source.
Power usage efficiency
There are various efficiency metrics one can use to rate a data center’s effectiveness. One of the most simple but effective measurements is power usage efficiency (PUE), which is the ratio between the total amount of power entering a data center by the amount of energy used by its IT equipment. It’s a simple way to measure how efficient a data center is. The most optimal is a ratio of 1.0.
Bytesnet has ratios as low as 1.20. This is a good score compared to other data centers in the Netherlands, which average around a 1.21 PUE and Worldwide, which average 1.58.
Conclusion
GenAI comes at the cost of significant energy. In a world in which a majority of energy sources are still fossil fuel based, it’s advisable to reduce consumption as much as possible. We listed some ways to do this, by avoiding LLMs and opting for SLMs when possible. Furthermore, it is recommended to use data centers which take care to implement green initiatives.
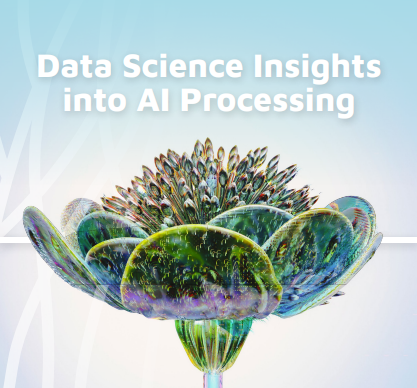
eBook
Download "Data Science Insights into AI Processing", the eBook for starting data scientists and analist, now for free.
eBook download
Fill out this form to download the eBook.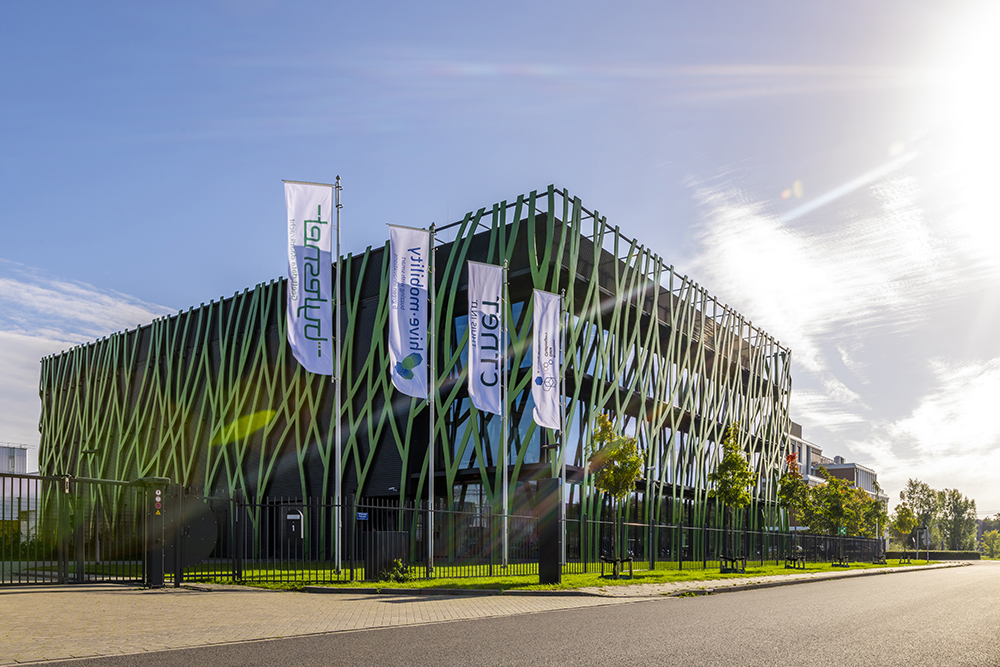
Contact us
Want to know more about GenAI? Then get in touch with us!